One of the major risks associated with a business is its inability to maintain sustainable growth. Failing to timely adapt to changes in consumer behavior, taste, and preferences can mean lost business opportunities and even worse, direct losses to a company. Predictive analytics, a subset of customer data analytics, allows businesses to stay one step ahead and grow in today’s highly competitive marketing space.
Although predictive analytics has been around for some time now, with advancements in technology and the growing popularity of Big Data, more and more companies are making it a part of their overall business analytics strategy. According to a report by The Insight Partners, the predictive analytics market is projected to grow from approximately $12.5 billion in 2022 to $38 billion in 2028 at a CAGR of 20.4%.
What is Predictive Analytics?
So, what is predictive analytics? As the term itself suggests, predictive analytics focuses on “predicting” future outcomes by analyzing existing data and identifying patterns and trends in that data. The goal is to prevent mistakes that can turn out to be costly and discover new growth opportunities. A variety of techniques that are used in predictive analytics include data mining, statistical modeling, machine learning (ML), and artificial intelligence (AI).
Predictive Analytics Models
Now, let’s have a look at the 5 different models used for predictive analytics.
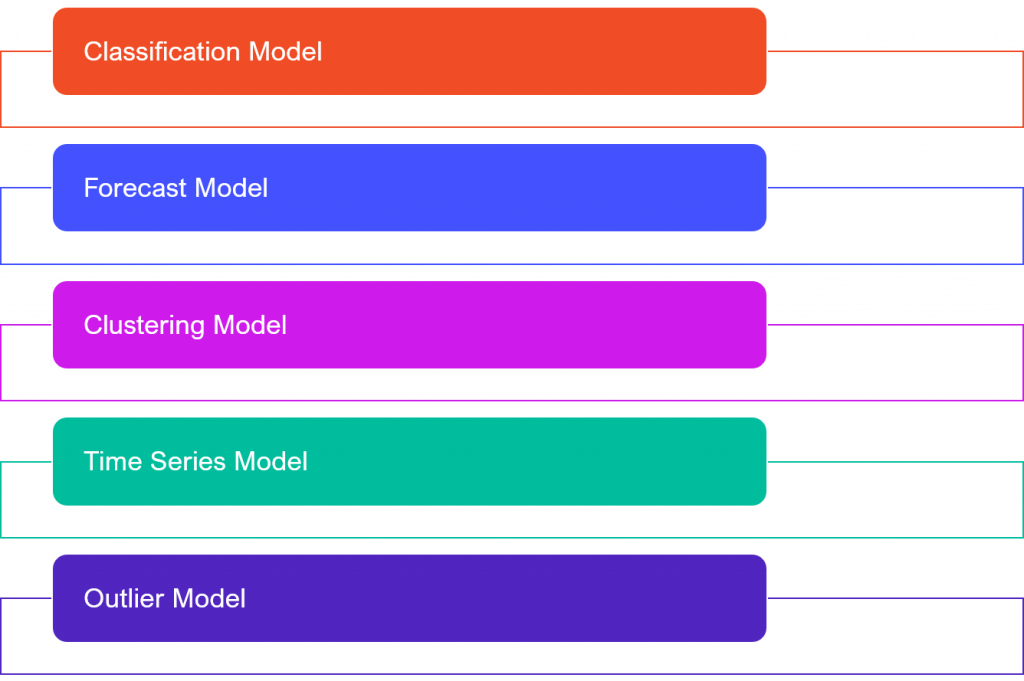
Classification Model
The classification model aims to broadly analyze historical data and categorize information by establishing relationships in datasets. This model is most suitable in situations where you’re looking to find answers to plain Yes or No questions. For example, “Will the customer switch to a relatively cheaper product if the price is increased?” E-commerce websites often use the classification model for product recommendations.
Forecast Model
The forecast model relies on learnings from historical data to estimate the metric value of new data with straight-line (linear) regression. It is typically used for large values and numerical data. Using the forecast model, the sales department can predict how many inquiries they will get in the next week, or a clothing store can predict how much inventory they would need for the sale day to entertain all orders.
Clustering Model
The clustering model is one step above the classification model. It focuses on combining data with similar attributes and putting it into separate groups using hierarchical clusters with decision trees. This predictive analytics model is particularly useful in targeted marketing where you’re looking to promote your product or service to a specific group of customers. The clustering can be hard or soft depending on the requirement.
Time Series Model
In the time series model, time is the input parameter. The model works by analyzing different data points in the historical data to develop metrics that will predict trends for a specified period. Using the time series model, a retail business can predict its next year’s sale based on the sale they did in the past three or five years. Because the model is based on linear regression, it can also be used for weekly, daily, and even hourly predictive analytics.
Outlier Model
Unlike the classification and forecast models that rely on historical data, the outlier model uses anomalous data entries within a dataset to derive a conclusion. Anomalies can happen if there is unusual data that is significantly different from the rest. The outlier model can be particularly helpful in fraud detection to avoid or minimize damage.
Key Challenges Faced by Retail Companies
Customer Loyalty
The idea behind maintaining customer loyalty is to make sure the customer keeps coming back to you—after a sale, which can be a big challenge. The more competitors you have, the more difficult it is to gain customer loyalty. Retail companies need to keep innovating to convince their customers that they are worth spending money on.
Effective Marketing
Knowing what to sell, whom to sell, when to sell, where to sell, and how to sell is of critical importance to a retail business. Not having an effective, data-driven marketing strategy in place can mean fewer sales and higher costs.
Compliance
Because retail companies gather a large amount of customer data, there are certain standards and government regulations that they need to comply with to ensure data safety. Inconsistencies in data collection, transfer, or processing can have legal implications for a retail company.
Data Silos
Siloed data is any data collected in a repository that is isolated or incompatible with other data sets, making its accessibility and analysis difficult for users in other departments. An outdated IT infrastructure or lack of effective data management can lead to data silos.
Why Retail Companies Need Predictive Analytics
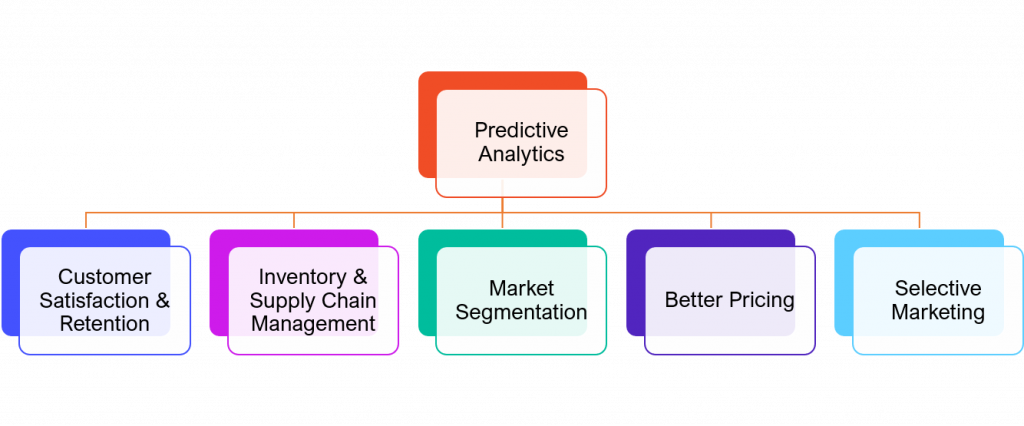
Customer Satisfaction & Retention
As they always say, “Customer is King”. Indeed, the success of a business greatly depends on how happy its customers are. Predictive analytics helps businesses in customer behavior analysis and understanding buying patterns. Based on their analysis, retail companies can come up with new loyalty programs, discounts, points, and reward systems to achieve maximum customer satisfaction and reduce churn.
Inventory & Supply Chain Management
As a retail store, the last thing you want is to have a high-in-demand product out-of-stock or a low-in-demand product available in bulk—as it can directly hamper your sales. With predictive analytics, you can get insights into what your customers need, at what time, and at what place, so there are no gaps between demand and supply.
Market Segmentation
Using predictive analytics, retail businesses can segment their customers into different groups based on various factors, such as their age, gender, interests, preferences, income, and demographics, also known as market segmentation. Market segmentation allows businesses to optimize their marketing campaigns and increase conversions and revenue.
Better Pricing
Wrong pricing can significantly influence the success of a product or service. A price which is too high might force shoppers to look for alternative options. Similarly, a price which is too low can be mistaken for compromised quality. Both scenarios are bad. With predictive analytics, retail businesses can figure out the right price for their products to generate more sales opportunities.
Selective Marketing
Market Basket Analysis (MBA) is a data mining technique that companies can use to find out what customers are most likely to buy. The aim is to do selective marketing by pooling similar products together for up-selling and cross-selling, The technique can also help in optimal product placement on a retail website to boost sales.
How Contata can Help You Drive More Value for Business with Predictive Analytics?
At Contata, we offer top-notch customer data analytics solutions for retail companies to help them drive more value to their business. We use a variety of predictive modeling techniques to enable our clients to:
-
- Gather more customer data, including contact details, demographics, etc.
-
- Identify and target potential customers from a large pool of users.
-
- Segment customers for targeted marketing and increase customer engagement.
-
- Find out “at-risk” customers and deploy retention strategies to minimize churn.
-
- Optimize resource utilization and boost ROI.
Our predictive modeling techniques include but are not limited to:
-
- Machine Learning
-
- Advanced Clustering
-
- Data Mining
-
- Lifetime Value Prediction
Conclusion
Predictive analytics is much more than just making assumptions. When done right, it can do wonders for a business. Nonetheless, not having enough data, and most importantly, good quality data, is a major barrier to organizations planning to use predictive analytics to their benefit. Having the right data analytics strategy in place is critical to ensure you have high-quality data to obtain critical business insights for strategic decision-making.
Know why your business needs a powerful data and AI strategy.